Leveraging Agriculture Dataset for Machine Learning: A Game Changer for Business
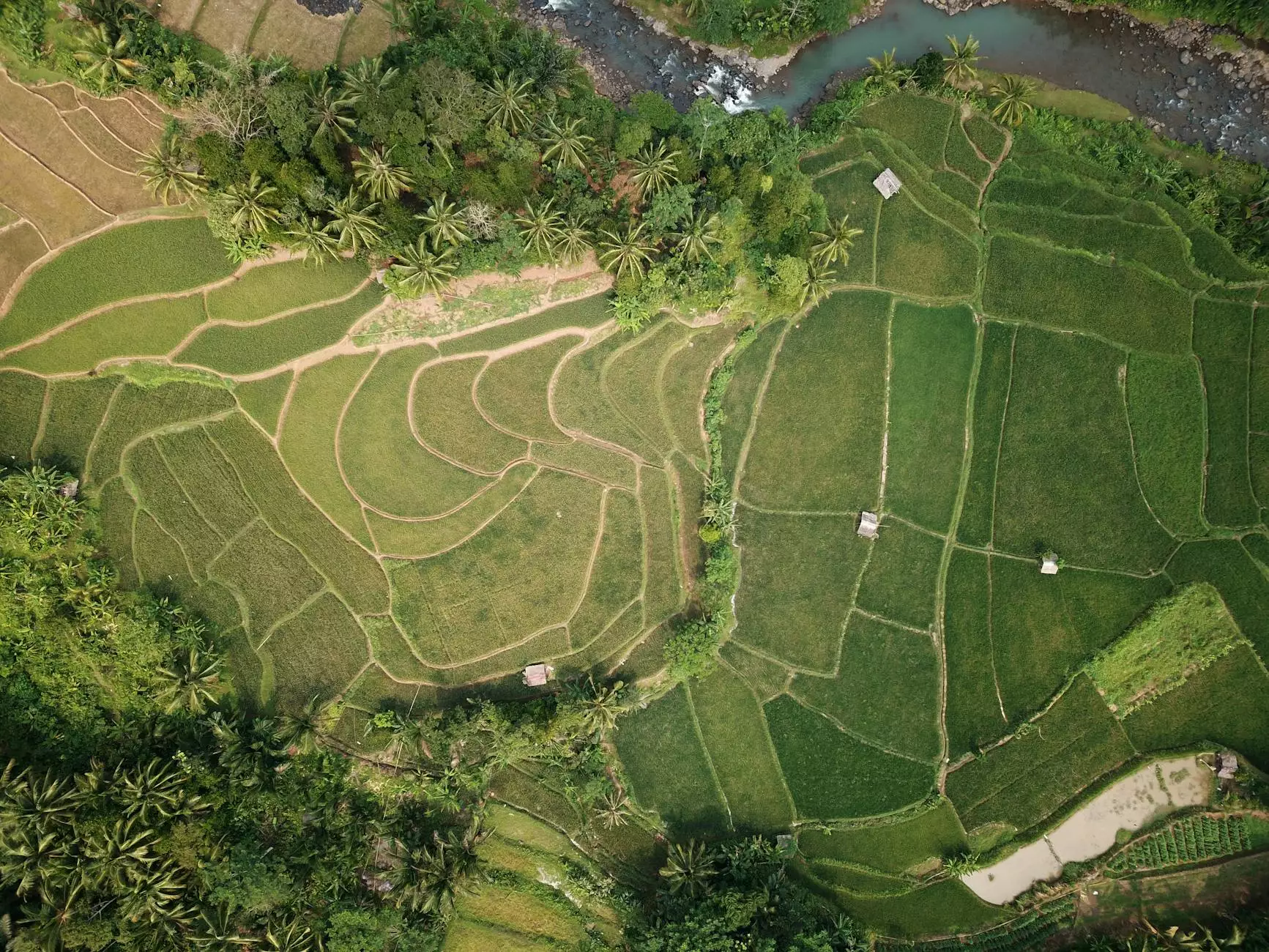
In today's tech-driven world, artificial intelligence and machine learning are becoming frontiers for all industries to explore. One of the most promising applications of these technologies lies within the agricultural sector. The availability of a comprehensive agriculture dataset for machine learning presents remarkable opportunities to revolutionize business practices in this ancient yet essential industry.
Understanding the Importance of Agriculture Datasets
Agriculture is foundational to the global economy, directly influencing food supply, market dynamics, and sustainability practices. By harnessing the power of data, businesses can achieve unprecedented insights into farming practices, crop yields, pest management, and more. The role of an agriculture dataset for machine learning cannot be overstated, as it empowers stakeholders to:
- Optimize Crop Management: Tailoring irrigation schedules, choosing optimal planting times, and selecting the best crop varieties.
- Enhance Yield Forecasting: Utilizing predictive analytics to forecast yields, thus optimizing logistics and supply chains.
- Improve Pest Control: Implementing machine learning algorithms to predict pest outbreaks, allowing farmers to take proactive measures.
- Facilitate Sustainable Practices: Promoting resource-efficient farming. Using data can help manage soil health, reduce waste, and promote biodiversity.
The Components of a Comprehensive Agriculture Dataset
To effectively utilize an agriculture dataset for machine learning, it’s crucial to understand its components. A robust dataset typically includes:
- Soil Characteristics: Information on soil type, pH levels, moisture content, and nutrient availability.
- Weather Data: Historical and current weather patterns, including rainfall, temperature, humidity, and wind speed.
- Crops Information: Data on various crops, including hardiness, growth rates, and resistance to different climates.
- Pest and Disease Reports: Records of pest occurrences, disease outbreaks, and their impacts on crop production.
- Market Trends: Insights into demand and supply fluctuations, pricing data, and customer preferences.
How Machine Learning Transforms Agricultural Practices
Machine learning algorithms applied to agriculture datasets result in multiple benefits, leading to more informed business decisions:
1. Predictive Analytics
With the aid of historical data and machine learning techniques, farmers can accurately predict crop yields and market trends. This predictive capability enables businesses to plan better and allocate resources more efficiently.
2. Precision Agriculture
Through technologies such as remote sensing and IoT devices, farmers can gather detailed analytics on crop health, soil conditions, and weather forecasts. By integrating these insights with machine learning models, they can apply fertilizers and pesticides more precisely, reducing costs and environmental impacts.
3. Automated Decision-Making
Machine learning models can automate various decision-making processes, including irrigation scheduling and pest management strategies. Automation reduces the time and effort required for manual interventions, leading to more efficient farm management.
4. Enhanced Supply Chain Management
Businesses can use data-driven insights to optimize supply chains by forecasting demand accurately, managing inventories efficiently, and improving logistics operations. An optimized supply chain ensures that fresh produce reaches markets in a timely manner while minimizing waste.
Real-World Applications of Agriculture Datasets
Several companies are already seeing the benefits of integrating agriculture datasets into their operations:
Case Study: Climate Corporation
Climate Corporation utilizes vast amounts of data to provide farmers with insights on weather patterns and agronomic practices. Their machine learning models analyze this data, enabling farmers to make informed decisions about planting and harvesting, thus maximizing their yields.
Case Study: John Deere
John Deere has implemented machine learning across its agricultural machinery to collect data on field conditions, crop health, and machine performance. This integration helps farmers optimize their machinery's use, ensuring maximum efficiency and effectiveness.
Data Sources for Agriculture Datasets
Businesses looking to leverage machine learning in agriculture must access the right datasets. Here are some valuable sources:
- Government and NGO Backgrounds: Many governments maintain databases related to agriculture, which are rich in historical data.
- Research Institutions: Universities and agricultural research organizations often publish studies and datasets valuable for machine learning.
- Open Data Initiatives: Websites like Kaggle and UCI Machine Learning Repository offer open-source datasets for various agricultural applications.
- Commercial Providers: Companies that specialize in agricultural data aggregation often provide comprehensive datasets for a fee.
Challenges of Using Agriculture Datasets for Machine Learning
While the rewards of using agriculture datasets are substantial, there are challenges to consider:
1. Data Quality and Availability
High-quality data is critical for successful machine learning applications. In many regions, data may be sparse, outdated, or inaccurate, impeding the effectiveness of algorithms.
2. Integration of Diverse Data Sources
Aggregating data from various sources—including soil samples, weather reports, and market prices—can be complex. Ensuring compatibility and consistency across datasets is vital for reliable analysis.
3. Skills Gap in Data Science
Many businesses in agriculture lack the necessary data science expertise to interpret complex datasets effectively. It's essential to either train current employees or hire skilled professionals who can bridge this gap.
Future Trends in Agriculture Datasets and Machine Learning
The world is on the brink of significant advancements in agricultural practices with the continued integration of machine learning. Some emerging trends include:
1. Increased Use of IoT and Automation
Connecting more devices to the internet will generate huge amounts of real-time data. This advancement will further empower machine learning algorithms to provide timely insights for agricultural management.
2. Blockchain for Traceability
With consumers increasingly demanding transparency in food sourcing, blockchain technology will work hand-in-hand with machine learning, ensuring data integrity and traceability from farm to table.
3. Advanced Weather Prediction Models
Improvements in machine learning models for weather prediction will enable farmers to react promptly to weather changes, thus minimizing risks.These prediction tools will become indispensable for real-time decision-making.
Conclusion: The Transformative Impact of Agriculture Datasets on Business
In conclusion, the enveloping potential of an agriculture dataset for machine learning is set to redefine conventional farming. The insights derived from structured data lead to enhanced productivity, improved market strategies, and sustainable practices. Businesses that adopt machine learning and leverage comprehensive agriculture datasets are poised to lead the way toward a more efficient and sustainable agricultural future.
The intersection of technology and agriculture is not just a trend; it represents a significant shift that can create immense value for businesses willing to embrace data-driven decision-making.